Artificial intelligence
As humans, we are very good at detecting subtle patterns in complex data. But individually we are limited in how much data we can consider. Machine learning can operate at tempos and scales that greatly exceed human capabilities, detecting relationships in data that humans would be unlikely to notice.
Lynker Analytics specializes in deep learning which is a field within machine learning that uses artificial neural networks to detect patterns in large data sets such as video or satellite imagery (computer vision). Our team develop tailored deep learning processing pipelines for large scale imagery data sets captured from satellites, airborne sensors, UAV (drones) and underwater (ROV) platforms.
We use open source machine learning frameworks such as Google’s Tensorflow framework to build repeatable neural network architectures to annotate, classify, segment and achieve object detection in imagery data. We can identify and design the right algorithmic approach to meet your needs.
For supervised learning tasks we have an advanced human in the loop active learning system which can accelerate project outcomes.
Active Learning is a machine learning training methodology used to achieve high accuracy models using only the most essential training inputs.
Our software componentry employs a human-in-the-loop methodology to iteratively select and present images for labelling. The neural network models propose labels on the images that have the largest entropy (least confidently labeled or most uncertain). This sampling method is unbiased and ensures only the most informative samples are reviewed and labelled by a human expert, leading to savings in human effort and processing time and more efficient datasets that can be used to improve the model.
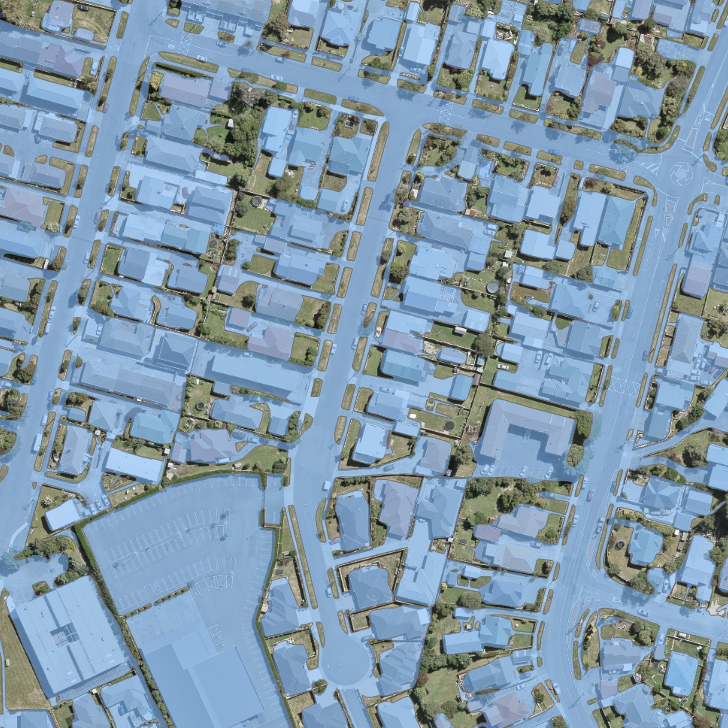
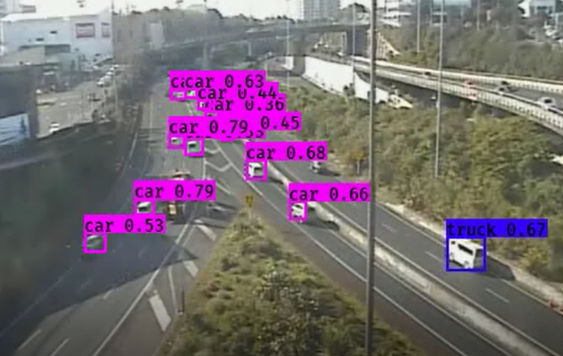
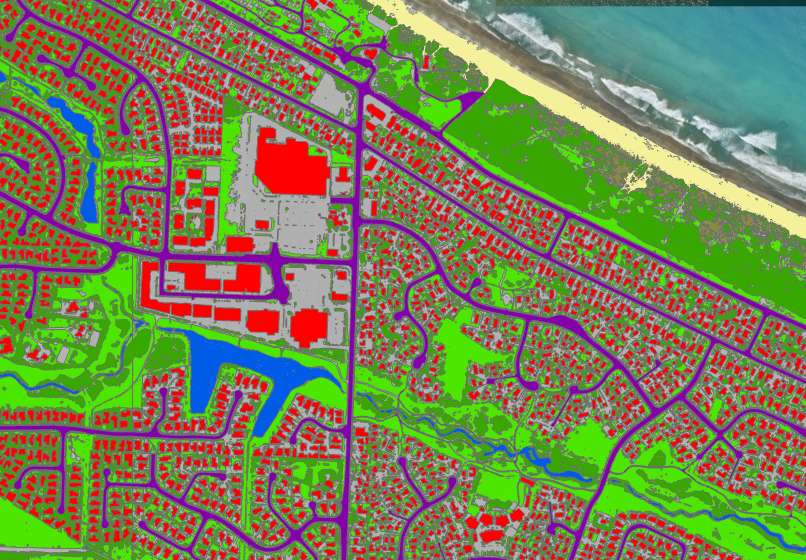
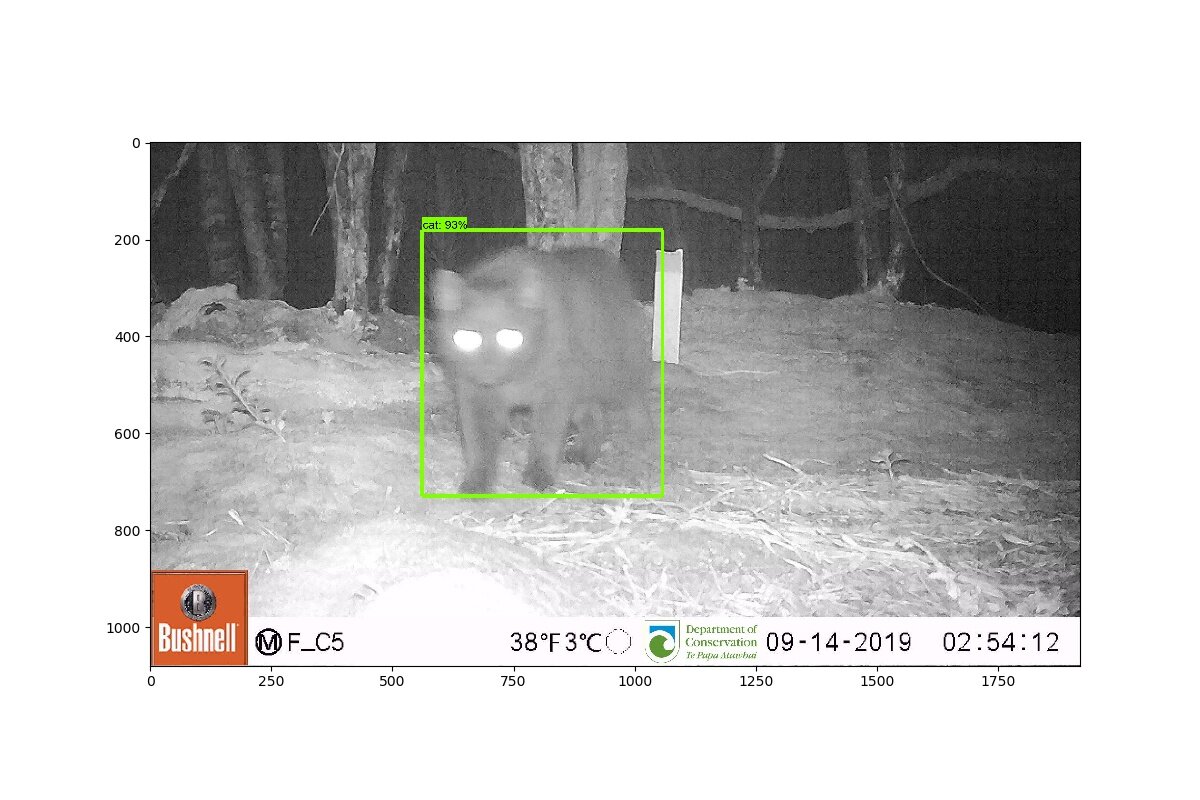
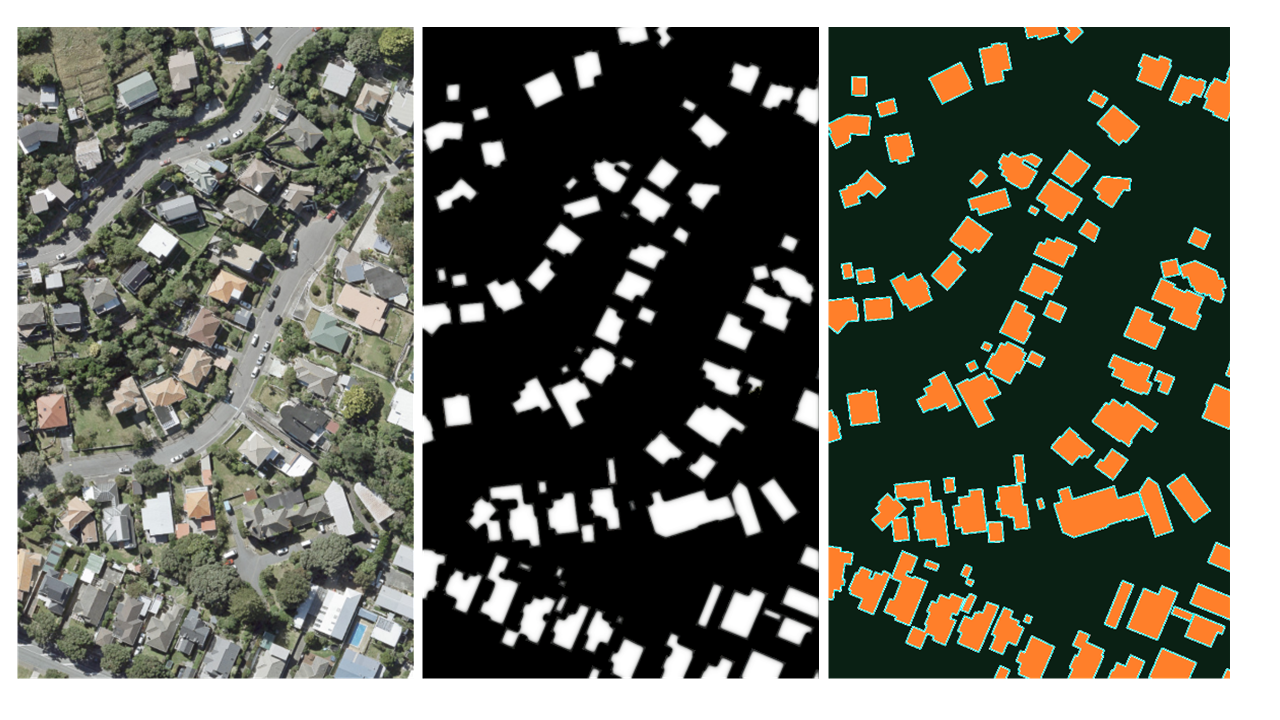
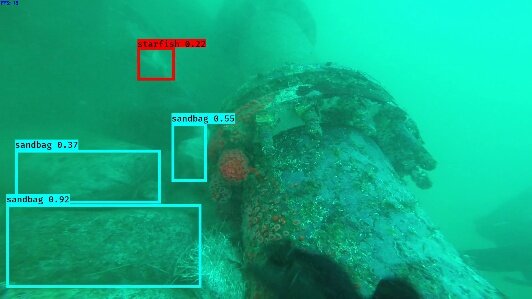
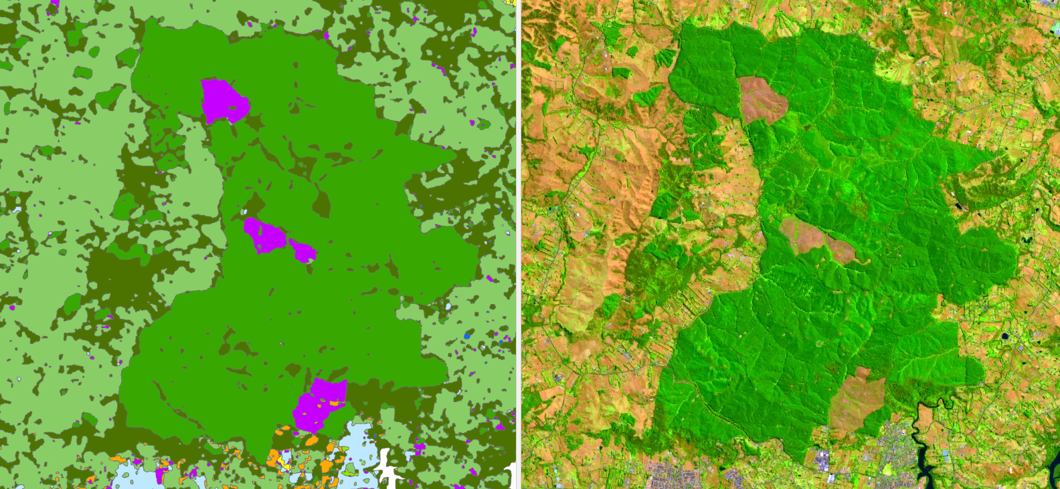
Our solutions are powered by adaptive and configurable web-based software designed with general purpose computer vision processing in mind. We have applied our methodology across multiple projects in a wide variety of fields
Environmental systems are almost always non-linear. Much of the data we gather about the environment contains unpredictable distributions, incomplete values or complex inter-relationships and feedback loops between our observed data sets. Intersect the natural world with the human built environment and things can get even more uncertain.
We use the inter-connected feature relationships held within a GIS to build location aware AI systems. This has a double benefit with AI systems benefiting greatly from GIS data streams while in turn geographic information systems themselves are the direct beneficiaries of machine learning by way of generating data with higher fidelity, frequency at a lower cost.